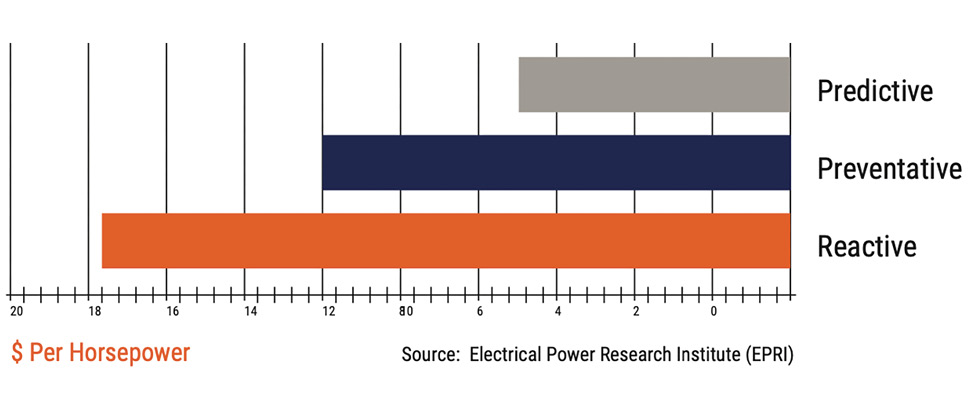
Industrial pumps play a crucial role in moving fluids and gases across various industries. They account for a significant portion of motor-driven equipment in industrial production facilities, contributing to substantial energy consumption. According to the United States Department of Energy (DOE), process pumps constitute 25% of total motor system energy use in production. Ensuring the smooth and reliable operation of these pumps can lead to significant energy savings, reduced maintenance time and lower repair costs. Predictive maintenance technologies are essential in achieving these benefits.
Reliable pumps are vital for maintaining continuous and efficient manufacturing processes. Without them, production halts, leading to unplanned downtime, which is a major concern for industrial manufacturers. Unplanned downtime costs Global 500 Industrials $1.5 trillion annually, with an average of 11% of revenues lost. For process manufacturing, the risks are even higher, potentially causing the loss of entire batches, as well as environmental hazards and safety issues.
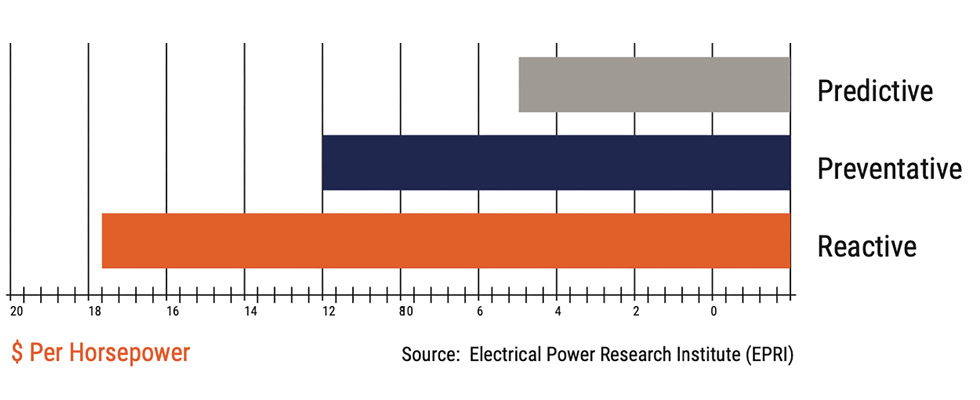
Remote monitoring that enables predictive maintenance is key to preventing unplanned downtime. It detects defects that could cause machinery failure—and does so early, accurately and automatically. Understanding the failure modes of pumps and utilizing sensor technology to detect these defects early is essential.
When pump parts develop defects, their reliability decreases, ultimately leading to failure. This results in downtime until repairs restore the pump’s functionality.
Pumps can encounter various failure modes, including hydraulic, mechanical, lubrication and electrical motor or electromechanical failures. A holistic approach using multiple sensors provides the best coverage for detecting these failure modes. Starting with the most probable and costly failure modes is crucial in the predictive maintenance journey.
Benefits of Predictive Maintenance
Predictive maintenance offers numerous benefits, particularly for industries reliant on pumps for critical processes. By understanding pump failure modes, deploying a range of sensors and utilizing a combination of physics-based and data-driven models, manufacturers can achieve accurate remaining useful life (RUL) predictions. According to a study by the DOE, a correctly deployed predictive maintenance program can have a return on investment (ROI) as high as 10X and can result in a 20 to 25% increase in production. The motivation for moving to a predictive regime is clear; the question is only how best to do it.
Transitioning to Predictive Maintenance
Vibration sensors are the most common sensors in predictive maintenance, but a comprehensive monitoring stack takes advantage of a broader suite of different sensors, the combination of which are tailored for the machine type in question driven by each machine’s common fault modes. Industry-standard alerts based on this sensor data can transition maintenance tasks from preventive (time- and usage-based) to predictive. For example, instead of changing oil based on time and usage, predictive maintenance allows for changes based on the actual condition of the pump, as noted by sensor monitoring. This approach extends the time between outages, thereby increasing equipment uptime and productivity.
Moving from reactive or preventive maintenance to predictive maintenance can significantly reduce maintenance costs. Data from the Electrical Power Research Institute shows that adopting a predictive maintenance approach can halve maintenance costs and reduce overall downtime.
Additionally, truly predictive maintenance provides more data for root cause analysis. For instance, rather than merely scheduling a roller bearing change, users can investigate why the bearing degrades faster than expected. Predictive maintenance practices help identify trends and causes, allowing for more proactive measures and further cost reductions.
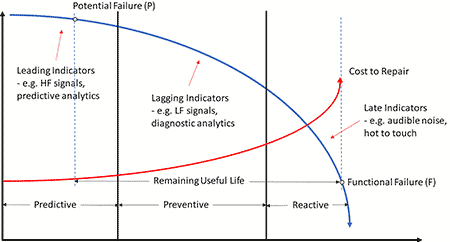
High-Frequency Sensors in Predictive Maintenance
High-frequency sensors—such as those measuring vibration, current or pressure—are critical in diagnosing failure modes and predicting maintenance needs. These sensors can detect failure modes like bearing wear, motor rotor bar defects and pump cavitation events much earlier than low-frequency sensors normally used for machine control. They enable accurate estimates of the RUL of pumps, allowing for optimal planning of maintenance activities.
Using high-frequency sensors, users can map out the degradation curve of an asset. The point of potential failure to failure (P-F) curve represents the degradation rate and highlights the benefits of early defect detection. Early detection can prevent emergency repairs, which can be up to six times more costly than planned maintenance.
Integrating Physics & Data-Driven Models
A failure modes, effects and criticality analysis (FMECA) study helps understand pump failure modes based on physical properties and operating environments. Combining sensor data, physics-based models and data-driven analytics improves the accuracy of predicting the degradation path and RUL. This integration enhances anomaly detection and forecast accuracy.
For example, vibration sensors can detect bearing defects, allowing users to plan maintenance tasks during convenient operational windows. Adding data-driven intelligence refines RUL estimates, providing a truer predictive maintenance solution. This improves confidence in making it to the next scheduled outage without unexpected failures.
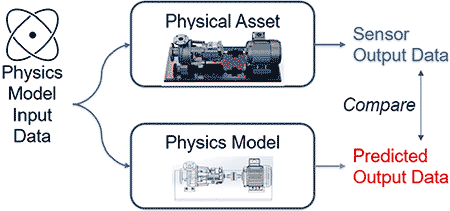
Remote Monitoring of Pumps
Remote monitoring enhances predictive maintenance by allowing continuous oversight of pump performance and enabling a qualified subject matter expert (SME) to review the system health without needing to be present on location. It enables early detection of defects and timely interventions, reducing unplanned downtime and optimizing maintenance schedules. Remote monitoring systems collect real-time data, providing insights into pump conditions and facilitating proactive maintenance strategies. This ensures pumps operate efficiently, saving energy and minimizing maintenance costs.
Predictive maintenance is essential for asset-intensive industries, particularly those dependent on pumps for critical manufacturing processes. By understanding various pump failure modes, employing a comprehensive sensor approach, and integrating physics-based and data-driven models, manufacturers can significantly reduce maintenance costs and improve reliability. Remote monitoring further enhances these benefits by enabling continuous oversight and timely interventions. Implementing these predictive maintenance practices leads to increased production output, reduced energy consumption and improved efficiency of plant personnel.