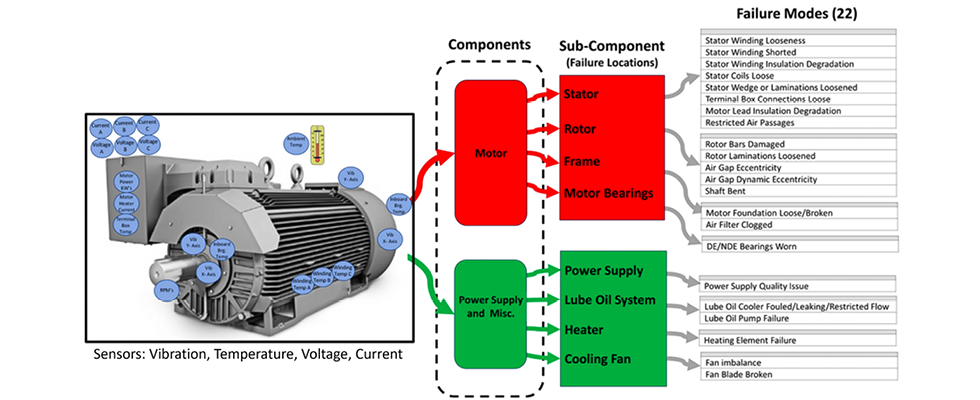
Business and operations systems are more integrated in this new digital era. With accessible data and information, it is easier to enhance the business value of plants that employ equipment to produce product. When equipment performance and availability are enhanced, production increases, fundamentally improving plant value.
Pumps play a vital role in process industries including chemicals, oil and gas, mining, water treatment and power generation. When pumps degrade in availability or performance due to a developing defect, production losses are experienced in downtime, performance losses and quality.
Pumps and systems are monitored in this digital era with a wide range of sensors to detect probable pump defects, automate analytics and recommend mitigating actions. Automating sensor data collection and analytics improves worker productivity by focusing the workforce on tasks that require human insight and decision-making. With automated sensor systems, benefits are accrued like lower maintenance costs, increased equipment life span, lower capital costs, increased production output, improved sustainability, reduction in safety incidents and improved visibility of pump health and performance.
This article will focus on the components of a digital predictive and prescriptive maintenance program and application. It will also describe the process of implementation and share a case study.
Components of a Predictive & Prescriptive Digital Maintenance Application
There are five categorical components of a digital predictive and prescriptive maintenance application (Image 1).
- The asset includes expected performance like electrical, mechanical and fluid dynamics; specifications including motor, coupling, pump type and replaceable parts; operating manuals and recommended maintenance.
- Sensors measure equipment performance and health, connecting digital systems to the electrical, mechanical and chemical world of the pumps. Predictive maintenance inspections include vibration, motor current, ultrasonic, thermal, lubrication, electromagnetic and video sensors. Process sensors include temperature, pressure, flow and chemical property sensors. The maintenance sensors and process sensors work together, providing a holistic view of the pump operating state, performance and health.
- Data acquisition digitizes the electrical signal from analog sensors, transforms digital sensor messages into numerical values and communicates with the data historian to gather digitized control system sensor data. The data acquisition system adds contextual information like sensor location, time stamps, units of measure, sampling parameters, data quality, operating state, etc.
- Analytics extracts features from sensor data. Features include statistical analysis (like rate of change), running averages and correlations with related pump sensors. Signal processing features like Fourier transform identify frequencies or tones in vibration, ultrasonic or electrical sensor data. Sensor data features map-to-pump degradation modes and provides a basis to set alarms and diagnostics.
- Decision support builds from feature analytics, informing plant personnel of any problem diagnosis with a recommended mitigating action. Rate of change and alarm levels provide a sense of severity and urgency when failure consequences are put in context. Knowing the plant operational schedule helps personnel target the least interruptive time to perform the mitigating action. At this level, subject matter experts (SMEs) review the recommended action and drill down through the analytics, analyzing raw sensor data and asset information. Feedback from SMEs influences analytics, data acquisition, sensors and the asset context.
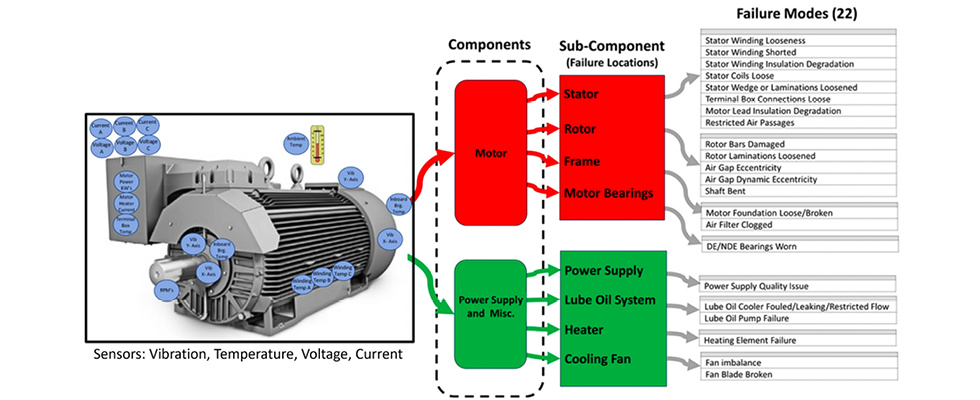
Putting the Components Together
Implementing a predictive maintenance program starts with the assets (pumps). Engineering documentation is fundamental to understand failure physics, performance expectations, failure consequences and respective mitigating actions.
Core to a pump predictive maintenance program is an asset catalog, the criticality or importance of assets to the business, probable failure modes and mitigating actions. These details are kept in an accessible, maintainable and digital location. The details are navigable by plant equipment hierarchy and by equipment type. Criticality and asset failure modes are most important (Image 2).
Knowing the failure modes and typical maintenance plans, a sensor survey identifies existing sensors and any gaps. Sensors are added to improve coverage of probable pump failure modes.
Data acquisition systems (DAQ) wire to sensor sources and installers configure sensors with location and context, including time, units of measure, data quality, etc. DAQs are provisioned on a network of choice, typically a virtual local area network (VLAN) with access to the plant data historian.
The DAQ transfers data to analytics. Analytics calculates features or pump condition indicators from statistics, correlations and Fourier transforms. The features combine and provide symptoms of pump health and performance degradation. The symptoms aggregate and form a probable diagnosis of the pump’s problem (Image 3). Decision support combines the current probabilities in its diagnosis with historical pump failures and corrective maintenance plans. With the diagnosis and successful actions in hand, the decision support system recommends a mitigating action to remove the defect, curing the pump of its problems.
Industry efforts to estimate remaining useful life (RUL) are ongoing. Defect severity and anomaly rate of change provide clues. In the future, mitigating action and RUL together ease corrective work scheduling with the least disruption to plant operations.
With pump engineering data, sensor selection, data acquisition and analytical feature extraction with diagnosis, the digital predictive maintenance system guides plant personnel in planning and scheduling mitigating action. SMEs drill down to engineering documentation, sensor features, diagnostics logic and original sensor values to validate and approve decision support recommendations.
Case Study: Duke Energy
In 2010, Duke Energy began a companywide program using digital technology to address reliability demands and optimize workforce activities.2 Duke replaced route-based data collection with DAQ technology to identify problems and notify SME specialists. SMEs now remotely spend time on higher value tasks. This multiyear project included new sensors and a new information technology architecture. Duke incorporated the industrial Internet of Things (IIoT) and big data analytics to meet its reliability goals. Building on maintenance and engineering knowledge of plant assets, Duke worked with the Electrical Power Research Institute (EPRI), sensor vendors, DAQ vendors, data historians and anomaly detection software. Duke’s vision was for fleetwide smart monitoring and diagnostics (Smart M&D).
EPRI introduced a similar framework to support power generation companies transitioning to IoT-related technologies and solutions. Duke and EPRI work together making investments in online sensors, networking infrastructure, system architecture and diagnostics applications.
Duke identified over 10,000 assets across their facilities and added more than 30,000 sensors to its equipment, including accelerometers, temperature sensors, oil analysis sensors, thermal cameras and proximity probes. These sensors add online vibration inspections, bearing temperature and oil pressure, transformers, dissolved gases and generator electromagnetic signatures.
Vibration inspections require captures up to 100,000 samples per second for several seconds to obtain good measures of machine condition. DAQs manage the acquisition, validate data quality, time-stamp data and extract features. To manage volumes of data, Duke uses edge computers. Every plant has its own data historian that collects, stores and organizes feature data. SMEs utilize alarm dashboards indicating unexpected equipment behavior and investigate screen issues to determine whether an anomaly needs further investigation.
Anomaly data transfers to the asset health management system for diagnosis by running a comparison against known faults in a fault signature database. The diagnosis provides a window to plan and schedule mitigating action at a time when costs are low. Over four years, Duke avoided costs greater than 130% of its capital budget by avoiding unforeseen failures. Automated data collection also reduced their labor costs.
Shifting to a digital predictive maintenance strategy improves reliability and lowers operating costs, meeting corporate reliability objectives while optimizing workforce activities with automation and analytics.
People and process must also be considered. The historic practice of using handheld analyzers for machinery inspections is an SME obstacle. Continuous improvement of remote visualization helps SMEs make the change to Smart M&D. Managing alerts is a challenge when 10,000 assets report anomalies. Incorporating criticality, severity and risk eases the alarm management challenge.
This digital era provides the tools and technologies to integrate engineering data, sensors, data acquisition, sensor feature analytics, statistics and machine learning into a holistic decision support predictive maintenance system. Implementation teams are grounded in equipment physics, plant production process, stakeholder needs and business process. It takes a team to deliver a successful implementation incorporating all five key components while managing organizational change.
References
- “Setting Up for a Successful Digital Transformation With Online Monitoring Technology,” National Instruments Webinar, 2022.
- “Duke Energy Leverages IIoT for Predictive Maintenance Applications,” IHS Market, Case Study, Alex West, January 2018.