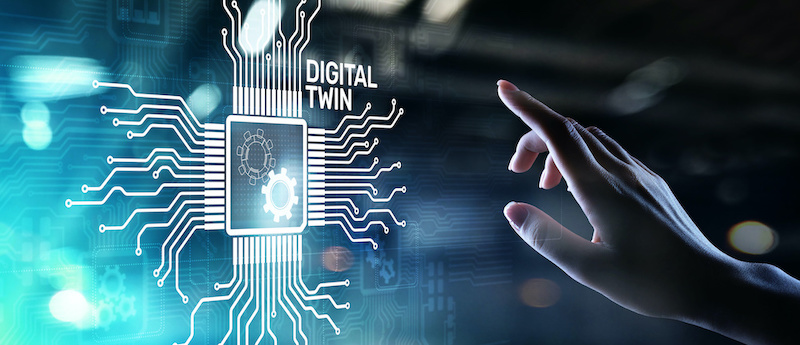
Internet of things (IoT) programs deploy digital twin concepts to make sense of complex data coming from pumps and valves. However, data without relevant context is redundant to existing systems, but multidimensional data represented by a digital twin can add value to enterprises.
This digital representation can provide insights into how physical assets are operating, how they have worked and how they could be managed better. Think of a digital twin as a three-dimensional object—key indicators and attributes, which are constantly updated. It contains a combination of dynamic sensor data, static asset data and performance analytical insights, all managed and represented as a single entity. Here are insights into an IoT strategy using digital twins to maximize pumps and valves.
The Full Pump System Picture
IoT provides the ability to detect changes in parameters on equipment such as pumps, valves, fans, motors and more. Many values can be analyzed to understand what, when and why things are changing. As teams find solutions for preventative maintenance and increased optimization, utilizing IoT to observe changes in equipment parameters such as vibration, surface temperature, moisture, pressure, speed, level and flow can save time and money.
Maintenance teams detect changes in equipment to understand why things are changing, but there is a lot of analysis that needs to occur to see the big picture. IoT and digital twins are the next steps as they allow these parameters to integrate to form the equipment digitally. Digital twins put those values together to create the equipment for users.
By laying out the data in a way that forms the equipment, it allows for faster decisions to be made. Therefore, there is less need to look at individual values to understand issues and correlate them. Digital twins combine these parameters so that users no longer look at if the vibration is high or low but how that value of pulsing correlates to pressure change. Those values together tell the history of the equipment and how each parameter affects the other. Digital twins also open up the option to add artificial intelligence (AI) to predict, analyze and assess equipment health.
Accurate Virtual Representation
A digital twin is a digital replica of a physical entity such as assets, processes, people, places, systems and devices used for various purposes. The representation shows how a pump, valve or piece of equipment lives through its life cycle.
First, this includes connecting the physical model and the corresponding virtual model or virtual counterpart. Second, the connection is established by generating real-time data using sensors. There are two distinct concepts in the definition of digital twins and in practice with two different use cases.
In the first part of the definition, a virtual model is used for computer simulation of the real-world system or device. The model is created to run as an executable model, driven by stimulus or various tests. The output of the simulation then produces data that replicates the corresponding real-world device. While this approach provides possibilities for testing, the output is only as good as the fidelity and accuracy of the model. The primary use for this approach is when users want to test numerous design options to see which is the most optimal.
The second key component of using digital twins uses sensors to represent the device, asset or system. The challenge here becomes having enough sensors to represent the entire asset properly. But the most critical part is the organization of data into an object-orientation.
The primary use for this second approach is where users have established, well-proven designs and a requirement to monitor the performance in the field. As such, sensors with digital twin object-orientation are the enabler for increased IoT-driven optimizations.
Key Benefits of a Digital Twin
Unlike real-world devices, implementation of the digital twin enables real-world machine learning algorithms to aggregate operational and performance data. Additionally, the data from the digital twin allows traceability, history and data analytics of the real-world device, thus making decision-making more data driven.
With the ability to monitor the behavior of physical pumps versus the ideal operating parameters, maintenance teams can compare all technical attributes and operational insights from similar equipment. Therefore, when starting with a well-thought-out data object, the digital twin analytics transforms the maintenance and management team’s work, providing a tool for comparisons, analytics, what-if scenarios and traceability.
Compared to simply collecting unorganized raw sensor data, the digital twin approach, when combined with the power of IoT, abstracts various sensor outputs into a digital twin framework, making physical assets come to life in meaningful ways.