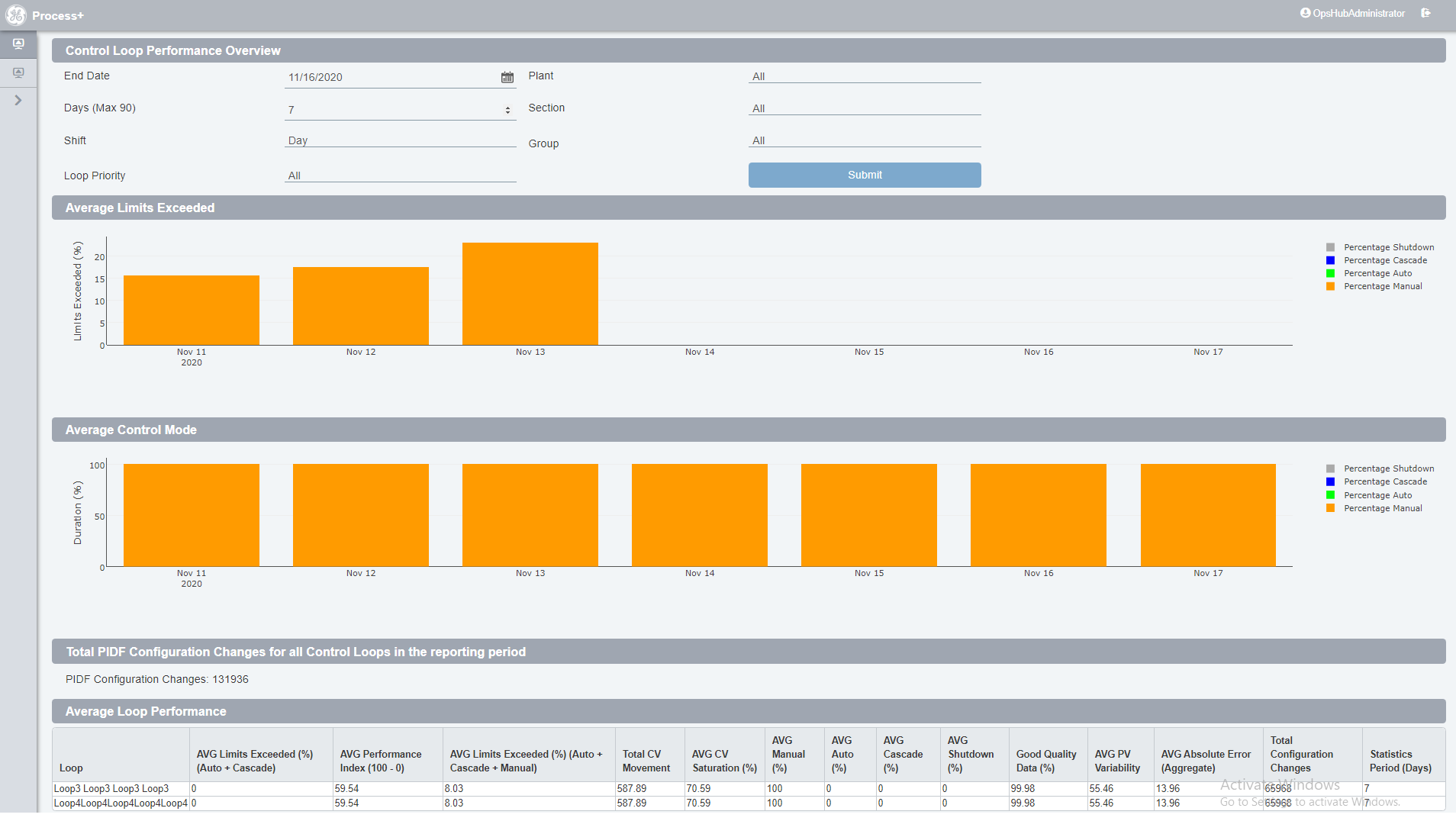
Advanced software techniques like machine learning and artificial intelligence (AI) used in predictive analytics play to an industrial process engineer’s strengths while taking advantage of domain expertise. With AI software tools, process engineers can identify risks, opportunities and future outcomes to better scale their operations. Additionally, while today’s software features enhance ease of use and no-code implementation extensible with programming languages like Python, process engineers can still lean on product experts in combination with their own domain expertise to mine data and leverage analytics to improve operations.
Recently, using AI-based predictive analytics software, a mid-sized water utility predicted pump failure up to 16 days in advance. This was achieved without writing a single line of code and using historical data that was readily available. The component identified as causing the pump failure was a critical bolt prone to corrosion—however, it was challenging to visually inspect based on its location. When the bolt eroded, its threads would loosen and lose contact, allowing the impeller to wobble. The extra vibrations created by this movement would result in more damage to the motor and its coupling. Eventually, the bolt head would separate, resulting in the impeller dropping out of the housing and causing a catastrophic failure.
Unfortunately, this inexpensive bolt takes this expensive pump out of commission for weeks. Using predictive analytics software and a trained data model, patterns and changes to vibration signals are now being monitored, and future failure can be detected. The water utility now has two weeks to schedule preventative maintenance versus wasting resources on unplanned downtime, resulting in only a one-day disruption versus weeks.
Value in Data
Water utilities have no shortage of data, real time and historical. However, getting value out of that data to ease compliance, reducing costs, and driving greater efficiency means using analytics as a foundation for optimization.
Fortunately, the journey to success with machine learning and analytics does not mean that process engineers need to be data scientists. Proven processes and software technologies make analytics doable for every industrial organization.
Process engineers have domain expertise to put together process models, or process digital twins, and be able to interpret the models. This is the foundation for improving competitive advantage and success with analytics.
To drive analytics and improve processes, engineers can align domain expertise to five capabilities:
- Analysis: automatic root cause identification accelerates continuous improvement
- Monitoring: early warnings reduce downtime and waste
- Prediction: proactive actions improve quality, stability and reliability
- Simulation: what-if simulations accelerate accurate decisions at a lower cost
- Optimization: optimal process setpoints improve throughput at acceptable quality by up to 10%
AI & Machine Learning Improves Optimization
Process optimization is key to water utilities, and control loops are the critical components. Out-of-tune loops can affect whether water meets specification, chemical usage and energy consumption and ultimately increase the risk of not being compliant. AI and machine learning can be used to improve and optimize control loops to generate big savings and reduce risks.
A simple form of a process controller is the thermostat which maintains the temperature of a room according to a given setpoint. It operates as a closed loop control device, trying to minimize the difference between the room temperature and the desired one. The industrial version is the proportional-integral-derivative (PID) control loop, an essential part of every process’s application. PID loops have been around for a long time. Loop controllers are available as standalone devices called single loop controllers, but the most common version is a piece of code that resides in a programmable logic controller (PLC) or a distributed control system (DCS). It makes it easier to combine them to create advanced control diagrams like cascade or feed-forward control, or split range required for complex control.
One example is dissolved oxygen control in the aeration section of activated sludge treatment plants that have a central impact on effluent water quality, efficiency of treatment and energy usage as well as the lifetime and wear on equipment such as blowers.
Analytics can be used to monitor and optimize the performance and tuning of PID control loops in the wastewater process (for example, dissolved oxygen control) to reduce process variation, compensate for disturbances and ensure the process operate close to optimal states. This can result in improvements in equipment life, effluent water quality and treatment efficiency and energy usage with margins.
For process optimization, analytics solutions need to provide multiple capabilities: process modeling and troubleshooting as well as online deployment and real time monitoring. When data is prepared, visualized and rules-based, data driven process models can be constructed. Using these models, root causes of process deviations are identified, so processes can be optimized.
Sensor Health Is Key
Applications for predictive analytics are endless, but as a possible first step: engineers can use analytics to monitor
sensor health.
Bad sensor data can mean chemical dosing issues, asset downtime, compliance issues and safety risks as well as a dirty data foundation for continuous improvement programs. Water utilities need to have good data that can be leveraged for operations, ad hoc analysis and higher-level analytics.
Over time, sensors tend to deviate, impacting processes and operations. It is time consuming to manually determine if and why sensors are working or failing ahead of increasing risk. Engineers can employ a predictive analytics app to continuously monitor and analyze sensor data. Users can target anomalies and minimize their potential impact. The analytics app provides an easy way to automate the detection of bad sensors, where data is deviating from normal conditions. When an anomaly is detected, the app can generate alarms to speed repairs, replacements and recalibrations. By using predictive analytics to monitor sensor health, engineers can:
- Reduce downtime: Sensors are often used to provide indications that equipment is running correctly. Incorrect readings can lead to equipment failure or damage. Early detection of a sensor that is no longer giving accurate or consistent results can provide advanced warning that enables maintenance to replace or recalibrate the sensor before the worst happens.
- Improve compliance: Sensors are often used for measuring whether the ambient surroundings of a process are within specification. If the sensors used are not accurate or not functioning correctly, it can lead to a process being out of specification and therefore introduce a risk of noncompliance.
- Ensure sensor data quality: Ensuring data quality in downstream analytics is part of internet of things (IoT)-fueled improvements. If the intent is more advanced use of analytics for a process, the need for ensuring data quality is critical.
A comprehensive analytic solution-development environment provides visual analytic building blocks to build and test calculations, predictive analytics and real-time optimization
and control solutions with connectivity to real-time and historical data sources and drag-and-drop access to rich, functional libraries.
Plug-and-play connectivity to historical and real-time data sources and automation systems make for faster configuration. Built-in support for data quality makes real-time data cleaning and validation easy.
Engineers should be able to save analytics solutions as reusable templates for easy deployment to similar assets or process units. Additionally, while the analytics troubleshooting component should enable engineers to find answers faster with analytics-guided data mining and process-performance troubleshooting, the development/configuration capabilities should allow them to more easily capture expert knowledge and best practices into high-value analytic templates for rapid enterprise-wide deployment.
All automation and process engineers can and need to develop capabilities in analytics and machine learning to remain competitive, both at an individual level as well as to help their industrial organization, in a world of digital transformation.
Over time, engineers can go from small projects to pilots to plant wide optimization with deep application of analytics. Engineers’ deep domain expertise provides a foundation for modeling processes and developing the analytics that are game changers in specific applications. The combination of applied analytics technology with those process twin models uncovers hidden opportunities for continuous improvement.