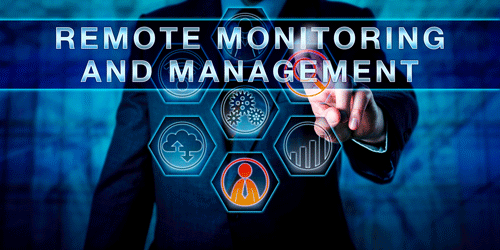
Pump, motor and fluid conditions that can be monitored are found across a pumping system, from the core of the system to the fringes. Sensors can detect a wide variety of these conditions. These sensors kick out lots of data, but they do not necessarily tell users what they need to know.
Place a conductivity monitor in a fluid system and take continuous measurements of the ability of that fluid to conduct electric current. Install a flow sensor in a pipe and take measurements of the movement of fluid in that pipe section. Mount a piezoelectric accelerometer to a pump and monitor the vibration signature of that motor. On that same motor, mount a thermocouple and detect the temperature of the motor casing. Doing all of this creates a number of data streams, feeding piles and then hills and eventually mountains of data.
Despite the volume of data being created in the previous examples, the data stream from any one of those sensors is not frequently the direct measure needed to make a decision. A key term in sensing and condition monitoring is inference. Why? That conductivity monitor is looking at just that—conductivity. The flow sensor detects movement within a portion of the fluid system. The vibration sensor detects the movement frequency of the portion of the motor to which it is mounted.
To reach a conclusion about the system requires evaluating multiple data streams in combination and applying intelligence and reasoning. Monitoring a sensing system tells the user exactly the conductance of the water in their treatment system. How useful is conductivity to a consumer of that water system? Not very. But that conductivity measure becomes quite useful if, from the raw data, an inferential conclusion can be made about the water purity.
Combining data increases the strength of an inferential conclusion. What if there is also a temperature sensor on the system? A pH monitor? Oxygen sensors? Sensors to monitor turbidity and total dissolved solids? That data, blended with the initial conductivity monitoring, can support high confidence inferential conclusions about the water quality in the treatment effluent.
Inferential monitoring can be about patterns. Link, combine and cross-connect the data to build a pattern of “normal,” then look for deviations from the norm. Baseline vibration data, collected from a healthy motor in a balanced system across a spread of frequencies and over time, tells what normal looks like for operation in that fluid system. Motor current data, collected over time and in those same conditions, paints an electrical picture of normal. The same goes for long-term flow data in the piping downstream of the pump. Send the pump into cavitation, however, and that motor current profile changes.
Motor vibration spikes in a couple of key frequencies. Fluid flow drops out of the normal band. All deviations can allow the user to infer the existence of cavitation. A different data set might lead to conclusions about a possible stuck pump rotor, and
from another, the user might infer they have a dry pump suction. The challenge for the control architecture is to recognize these deviations, identify the anomalies, make inferences and act to correct or protect the system. Motor monitoring to determine motor wellness is another area ripe for inference. All that vibration data gathered in the earlier example is just that—data—until it is charted, plotted, combined or compared to tell something meaningful about the motor’s state.
At first, it may seem to be just a frequency plot. Combine that data with a bearing temperature monitor, current sensor on the motor power feed and a flow sensor in the discharge piping, however, and intelligent and reasoned conclusions can be reached about that motor to make a decision: to continue to operate, or to take it offline and service it to prevent a catastrophic failure.
These data streams, and the inferential analyses needed to reach conclusions about and make decisions on a system, are well suited to artificial intelligence (AI) and machine learning (ML) algorithms. Those algorithms can hone the data, identifying and matching patterns to organize and prioritize decision criteria and improve the quality of system output—while also giving advance warning of issues that might take the system down.
Feedback from an AI/ML journey will likely inform decisions on sensor selection and placement—for example, moving sensors upstream to further develop leading indicators of system conditions and thereby improve system responsiveness. Describing a move “upstream” in the system does not always mean moving from the discharge to the suction side of the pump to monitor conditions farther away from the physical effluent stream. It might mean a move to monitor the electrical power feeding the pump in addition to the monitors that have been placed in the flow stream itself. Current sensors can be placed in the electrical feed to the motor and are leading indicators, signaling a change in parameters long before communicating a change in the fluid environment.
Data from sensors in a fluid system comes in a fast stream. Parameters monitored only tell a user so much, and inference is the key to reasoned conclusions—and to smart and timely decisions from a control system.