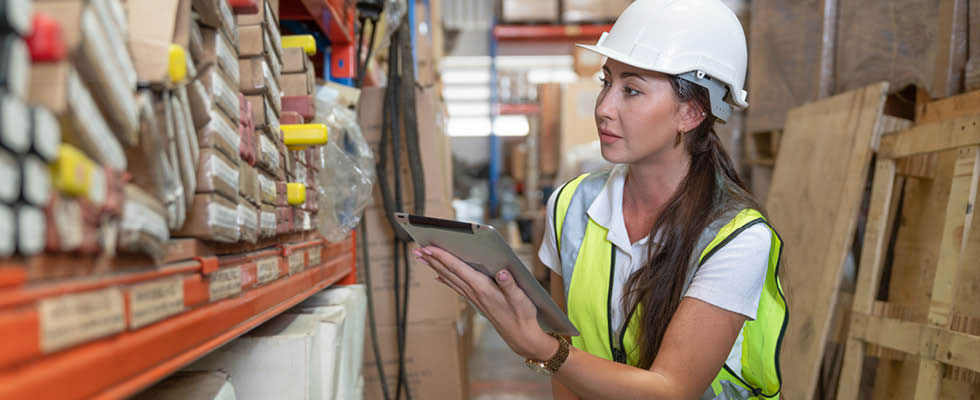
Today’s maintenance managers face a Herculean challenge. They must keep a complex array of assets up and running, all without exceeding the budget. In many cases, maintenance teams are also operating with a shrinking workforce and severe expertise constraints. Consumer demand and tight production schedules mean managers are under constant pressure to achieve more with fewer resources.
Shifting to a predictive maintenance program has been a game changer for many organizations. Predictive maintenance allows teams to harness the power of data analytics to predict machine faults and mitigate them, long before they lead to asset failure. The result? A sharp increase in productivity and uptime and a drop in maintenance costs.
Still, many managers today are struggling to implement a predictive maintenance and condition monitoring strategy. Perhaps this sounds familiar.
While every operation is different, there are a few common obstacles. Stakeholders may be concerned about the financial cost of predictive maintenance, managers may be uncertain about the training and implementation stage or they may simply be at a loss for where to begin.
In this article, I will explore these common obstacles and discuss how to overcome them. I will also discuss the concrete steps needed to shift from a reactive maintenance strategy to a predictive approach.
What Is Reactive Maintenance?
Reactive maintenance is the default maintenance strategy for many operations, especially those that are stretched too thin. Reactive maintenance operates on the principle of wait and see, delaying repairs until an asset finally breaks down. This is also known as run-to-failure. On the surface, it looks like a cost-cutting strategy. After all, you are only repairing or replacing assets when they absolutely need it; however, run-to-failure wastes valuable resources in most cases. The approach can lead to costly lost production time and reduced product quality. It also means more time-consuming and expensive repairs. By turning maintenance teams into firefighters, reactive maintenance also takes their time away from other important activities like effective inventory management and scheduling.
Let’s be clear: Reactive maintenance does have some built-in advantages, and it has its time and place. Reactive maintenance can be an effective short-term or backstop strategy if assets do fail. Run-to-failure can also be an effective approach for unrepairable items or for non-critical assets, but a reactive strategy is not effective for maintaining the key assets in a modern plant.
What Is Predictive Maintenance?
Predictive maintenance is a better way to manage your critical assets, allowing you to make smart maintenance decisions based on condition data from the assets themselves. Predictive maintenance leverages streams of condition-monitoring data captured via wireless sensors, handheld tools, supervisory control and data acquisition (SCADA) systems and more, combined with modern software and analytics. The combination of real-time condition-monitoring data and advanced analytics makes it possible for maintenance managers to predict and prevent machine failures before they occur.
Today’s software can scan condition monitoring data for signs of a new or developing machine fault. Changes in vibration or temperature patterns, for example, are often early warning signs of a more serious problem. When these changes are identified in time, technicians have ample opportunity to correct the issue so the plant can continue its normal operations without the costs, hazards and productivity disruptions associated with unplanned downtime.
Common Roadblocks to Predictive Maintenance
What prevents more operations from implementing a predictive maintenance strategy? Factors like budget and expertise constraints can play a big role here. Managers trying to make the most of their resources sometimes balk at the idea of buying new tools or investing in a computerized maintenance management system (CMMS). In a similar way, some managers worry they do not have enough skilled workers to implement the approach.
These are understandable concerns, but in fact, the nature of predictive maintenance makes it a great fit for plants on a lean budget and for operations with few skilled workers. There are a wide variety of condition monitoring tools available today, from wireless sensors to handheld devices, that are accessible for virtually any team or budget. Cloud technology also makes it easy to bring in global experts to advise expertise-constrained teams. High-quality CMMS software can guide decision makers across multiple worksites.
It is worth stressing that predictive maintenance is not a one-size-fits-all solution. The approach can be customized, modified and built out to meet the needs of virtually any operation. When it is done successfully, predictive maintenance maximizes resources and avoids costly downtime, more than making up for any initial cost involved in the setup. In addition, maintenance teams can and should start with a small pilot program, monitoring just a few assets at first, to prove out the time- and cost-savings before implementing a full predictive maintenance program.
It is also worth adding that not every asset in the plant needs to be monitored with sensors. One of the first steps in implementing a predictive maintenance strategy is creating an asset hierarchy and an individualized plan for how to keep each piece of equipment up and running.
Let’s get into the actual process of shifting from reactive to predictive maintenance. It breaks down into six
key steps, with room for customization at any point.
The 6 Steps of Implementation
1. Conduct an asset-criticality analysis.
An analysis of your assets’ criticality is key for determining a successful maintenance plan. Assess each piece of equipment according to its importance to the organization. It is a good idea to consider not only an asset’s impact on production, but also the impact of its potential failure. It is also a good idea to determine the asset’s impact on other key pieces of machinery.
2. Build a pilot program.
Begin by identifying a small set of key assets. Determine what the most common failure modes for those assets are and what form of condition monitoring data would be most appropriate for detecting those failure modes. Next, decide on the best equipment for gathering that condition monitoring data, based on your budget and available labor. It is also important to decide on the right data analytics tools, whether that means partnering with a third-party expert, using software or hiring a data scientist.
3. Establish benchmarks and evaluation criteria for your pilot program.
Determine criteria for a successful pilot and methods to evaluate the program’s success. Set goals, such as a number of correctly diagnosed machine faults over a fixed period of time or perhaps a specific shift in asset performance. You may also want to set a threshold for errors, like misdiagnosed or missed machine faults.
4. Begin the pilot phase.
Set a clear timeline and decide on rules like how frequently you will collect data and how your teams will respond to the condition monitoring data. It is vital to share the plan, the benchmarks and the ongoing process with all the relevant team members and decision-makers. Teams should have a strong understanding of the pilot’s operating procedures, goals and mechanisms.
5. Assess the results.
At the conclusion of the pilot program, review the results with your team and relevant decision-makers. Which aspects of the program were successful? Which areas showed room for improvement? The review process is crucial for implementing a new strategy and for continuing to improve.
6. Expand the pilot program.
Grow your predictive maintenance program incrementally and intentionally. After the pilot program, expand the program to another group of critical assets, using the lessons learned to improve on your implementation. This process should continue at a steady cadence.
Looking Ahead
Today’s predictive maintenance tools can achieve amazing results—especially when teams pair sensors with a top-of-the-line CMMS. Together, these tools can predict machine failures before they happen and issue alerts so maintenance teams know exactly what needs their attention. A CMMS can even generate
work orders and schedule tasks automatically, so your maintenance team never misses a beat.
The future promises to deliver even more. Artificial intelligence and machine-learning technologies are poised to sharpen diagnostic capabilities so they can pinpoint the root causes of machine failures and recommend solutions. Experts call this approach prescriptive maintenance or prescriptive analytics.
Operations that successfully adopt today’s best tools will be well-positioned for the technology of tomorrow. Technology never stands still. It is time to get ready for the workplace of the future by onboarding the best technology available today.