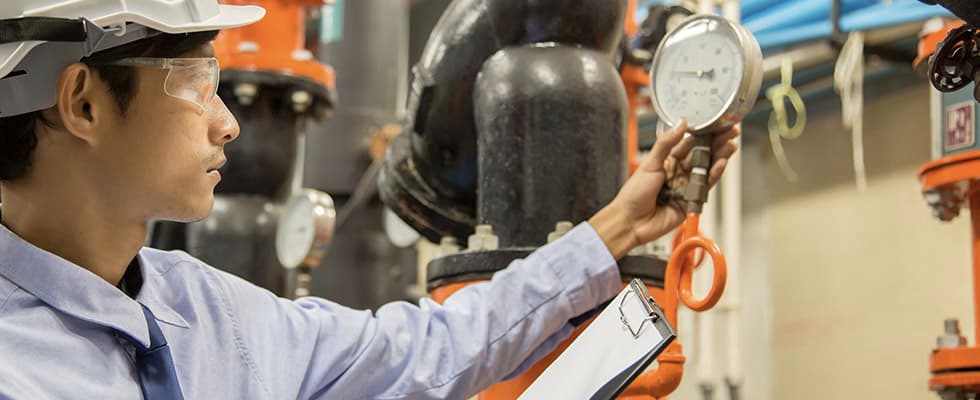
Unscheduled shutdowns, failure, maintenance and part replacement are key factors of operation, efficiency and economy for any plant or facility. About 20 to 40 percent of overall operating costs are due to maintenance actions, which can be a considerable amount of money. A key factor for improvements in maintenance policies, preventing failures, and avoiding unnecessary part replacement is the ability to predict the remaining useful life (RUL) of machineries and their key components.
RUL prediction is challenging and difficult, just as any branch of science/engineering for future prediction (such as weather-forecasting) can be error-prone and challenging. The estimation of remaining life is usually considered a theoretical topic and deals with extensive mathematical formulations and numerical simulations. This article discusses practical guidelines for predicting the remaining life of pumps and their key components.
Unscheduled Shutdowns & Scheduled Maintenance
Scheduled shutdowns are costly and should be delayed as far as safely and reliably possible. The time intervals between overhauls should be long. Unscheduled shutdowns can be expensive and troublesome and should be avoided.
RUL prediction is an important step to avoid unscheduled shutdowns and delay the scheduled maintenance or part/pump replacement. This prediction can have a positive impact on the overall operation, reliability, safety and economy of the production. This capability can result in lower operational costs, higher availability and optimized operational time intervals between part replacement actions or major overhauls. Responding to these predictions with timely maintenance actions helps avoid catastrophic failures or unnecessary repairs/part replacement.
Prognosis & Remaining Life
Prognostics is an engineering discipline focused on predicting RUL. Prognosis is the knowledge of the future condition of equipment and machineries. Usually performed through sensor signals monitoring, prognosis is closely related to diagnosis and condition monitoring. The final outcome of prognosis is the knowledge of the remaining/operational life.
A good knowledge of modes of failure of the pump and its key components is essential in developing the remaining life estimations for them. Unless the degradation/wear mechanisms are understood, no step toward reliable condition monitoring and estimation of remaining life can be taken.
A shortfall in many plants is condition monitoring data from pumps (and other machineries) have not translated into enhanced fault diagnosis, good detection/identification, prognosis and remaining-life/operational data. There have been considerable amounts of condition monitoring data (vibration, performance monitoring, oil analysis, etc.) in each plant or facility stored with no analysis to find rate of changes, diagnosis or prognosis.
Multiple/Independent Monitoring
RUL estimations usually depend on the accuracy of the monitoring sensors and fusion of monitored data. The prediction of remaining life is closely related to condition monitoring and data collection using multiple and independent sensors (monitoring methods) such as vibration monitoring, lubrication oil analysis, wear debris monitoring, acoustic emission (AE) monitoring and others.
A single condition monitoring method might be misleading. However, if multiple, independent monitoring methods indicate similar results and lead to similar predictions, such produced life predictions are more reliable. In other words, prognosis is linked with the use of multiple sensors, monitoring and fusion of data. The automation aspect of data study is related to digital signal processing and machine learning techniques.
Phases of Degradation, Wear & Damages
If there is a serious problem in a pump, its key components, assembly or installation—such as an incorrect seal selection or installation—it will usually show up in startup or in the first weeks of operation. If a pump has operated successfully for the guarantee/warranty period (say 12 months or 18 months), then degradation, wear and damaging mechanisms will be the most likely reasons for possible failures. In many degradation, wear and damaging mechanisms, following an initial nonlinear phase, there are two phases of wear development:
- A linear phase, with an almost constant rate.
- A short, nonlinear terminal phase where the wear/damage rate increases exponentially. This is an explicit indication of an imminent failure.
Transitions & Rate of Changes
There are usually subtle shifts (transitions) from “normal operation” to “defective operation” and, finally, failure. The rate or speed of change is important in this regard. Monitored or measured parameters can be categorized as:
- slowly varying parameters
- fast varying parameters
A key factor is how fast a parameter—such as vibration, leaked fluid through seals, an oil parameter, drop in head or drop in flow rate—is changing from its baseline to its alarm set points (or other defined limits). It is important to assess how fast a parameter changed from its normal value to the defined limits. This rate of change is usually more important than the normal/baseline or limit.
Often in the case of concerning degradation or developing damage (or in nonlinear terminal degradation/damage), change is relatively fast. In the case of normal and slow wear/degradation (or in linear low-speed wear/degradation), the speed of changes is usually relatively slow.
Data-Driven Life Prediction Methods
Various data-driven methods have been proposed for predicting the RUL of a degrading pump or degrading components of a pump (the time left before failure). Data-driven methods are of interest when an explicit model of the degradation process is not known. They are based on observations of the degradation/wear process of one or more similar pumps (or similar components), and usually perform the regression of the future degradation path until predefined criteria of failure are met. This is based on predicting the remaining life of a degrading pump/component and providing a measure of confidence on the prediction based on a set of reference degradation trajectories experienced by similar pump/component failure in the past.
Two key elements in the application of this group of methods are:
- How strong is the desired interpretation of similarity?
- What degree of trust is given to the reference degradation trajectories?
Among data-driven methods, there are two main subcategories:
- Degradation-based (wear-based) approaches. These methods model the degradation/wear evolution. These are statistical models that learn the degradation evolution (wear, damage, etc.) from time series of the observed degradation/wear states. The predicted degradation/wear state is then compared with the failure criteria.
- Direct life prediction approaches. These methods directly predict the RUL. These are typically artificial intelligence (AI) techniques that directly map the relation between the observable parameters and the remaining life, without the need of predicting the degradation/wear state evolution toward a failure threshold.
Degradation-based prognostics provide more informative and transparent outcomes than direct remaining-life prediction prognostics, since it supplies a prediction not only of the current RUL, but also of the entire degradation trajectory.
However, degradation-based prognostics require identifying a degradation indicator and fixing a failure threshold, which may be difficult in practice, especially in cases where few or irregular degradation trajectories are available and may introduce further uncertainty and sources of errors.
Challenges & Uncertainties
The remaining life estimates of pumps should consider intrinsic uncertainties due to the variability of the degradation, wear and damage processes caused by an array of factors, for example, by unforeseen future loads, operational settings, external conditions and more.
A major source of uncertainty is randomness in the future degradation/wear dynamics of the pump. This randomness stems from changes in parameters, such as head, flow rate, load, temperature or the appearance of a sudden failure.
Another challenge is the scarcity of information typically available about RUL of pumps along with associated wear, degradation and failure. Actual failure histories can be difficult to obtain. The exact replication of such events in a laboratory is insufficient by nature, often expensive, difficult and time-consuming.
To bypass these problems, simulations may be proposed. The framework of such simulations should be based on real-life experiments. However, there are many challenges, difficulties and inaccuracies associated with those, too.
There are many wear, degradation, and damaging mechanisms—such as fatigue, corrosion, erosion, creep and crack-developing—acting on each part or component and some interact with each other. Many degradation and wear processes are nonlinear and depend on many factors, parameters and conditions. There are numerous parts and items in each pump. They, too, can interact with each other. The complexity of the pump and the complications in the operating environment make it hard to establish a set of reliable processes for remaining life estimations. All of these can complicate the matter further.
Comparisons of identical pumps may be proposed. However, there are still challenges. Two identical pumps in the same service may not experience the same degradation and wear path.
Considering the history of operation, these so-called two identical pumps did not experience the same degradation and wear path up to the present time and will not keep following the same path in the future.
In general, there are uncertainties in current, past and future degradation/wear. Considering all the above-mentioned difficulties, ambiguities and errors, uncertainty management becomes a fundamental task in the prediction of remaining life.