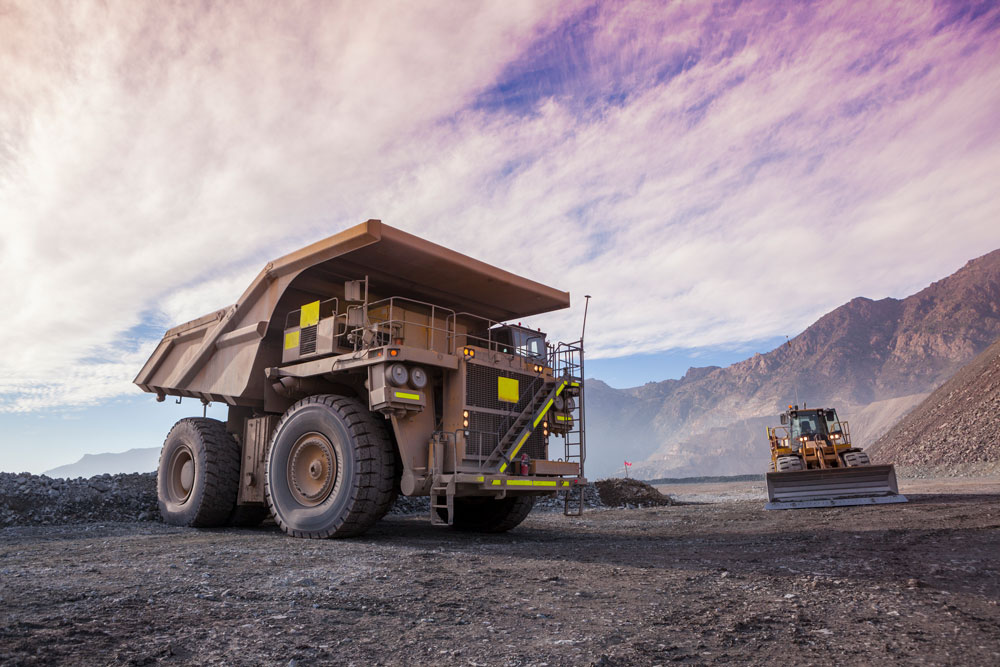
The majority of mining operations run 365 days a year to meet customer demand. Therefore, uptime of mining equipment is critical. Any unplanned downtime can dramatically impact productivity and profitability. There are many assets used for mining operations, including excavators, dozers and dump trucks. Dump trucks used in mining are critical vehicles necessary for the transportation of material across a mining site. It is important that these trucks, which run along the same path back and forth, day and night, are in working order at all times. Vehicle failures can put some trucks out of commission. This leads to stoppages in material removal from the site and/or materials not reaching their destination at the right time, resulting in unexpected delays and increased costs.
To avoid these vehicle failures, many companies increase preventative maintenance and create regular inspection schedules. These practices can lead to a decrease in productivity and return on investment (ROI) due to unnecessary part replacement, maintenance costs and labor. Others may rely on condition-based maintenance, which focuses on maintenance performed after monitoring real-time data and detecting unacceptable condition levels. However, this detection may not come with the advanced warning needed to prevent impending failure or avoid downtime.
By taking a predictive approach, past maintenance data and current sensor measurements can be used to determine early signs of failure before it occurs. This allows users to perform necessary maintenance only at the exact time it is needed, preventing both unexpected downtime and unnecessary maintenance.
Challenges in Deploying Predictive Maintenance for Industrial Vehicles
Determining the right health value threshold from vehicle data is not trivial or easy given the volume of signal tags collected (temperature, pressures, currents, voltages, rotational speeds) and the variety of operational modes/states in which an industrial vehicle can operate. For example, a higher exhaust temperature could be expected if the vehicle is operating in a warmer ambient environment and if the engine load is higher. In this case, the use of machine learning is needed to review multiple signals at the same time and determine the expected sensor value/behavior as compared to using fixed thresholds and limits.
Controller area network (CAN) bus data can be used in health monitoring; however, it is typically noisy and not sensitive to all faults, specifically misfire or combustion-related faults, which are difficult to detect with this lower frequency data. In this case, it is important to outline which failure modes are the most critical and determine if the CAN bus data would be sufficient for those failure modes or if additional instrumentation would be needed to achieve the desired accuracy for a predictive maintenance solution.
Data transmission in mining applications is also a challenge in terms of local storage or processing power that is available on the vehicle. Typically, it is not feasible to transmit all the raw data back to a central remote monitoring center. Local data storage and onboard edge analytics are needed to initially capture and process the data. This processed data is then typically sent back to a remote monitoring system. More advanced machine learning and pattern recognition analytics are conducted within this system to estimate the health condition of the monitored vehicle and its subsystems.
Harsh environments also present challenges in data collection. Additional requirements are needed to ensure the reliability of the sensors and hardware for the vehicle monitoring system, as the equipment has to withstand the same environment as the vehicle itself. These environments can also lead to more accelerated rates of degradation for vehicle components, given the environment and heavy loads that a vehicle experiences, making health monitoring efforts more difficult.
Case Study: A Predictive Maintenance Solution for Mining Trucks
An international mining equipment manufacturer had made progress in recent years delivering condition-based monitoring technologies and services to its clients in the mining industry; however, unexpected failures still occurred, resulting in costly interruptions in operations.
By working with a predictive analytics company, this client sought to address the issues experienced by its users by integrating predictive technology with its own advanced health monitoring offerings.
During this engagement, the predictive analytics company reviewed the maintenance records and historical fault data from 12 different mining machines, as well as sensor measurements with a total of 45 different parameters. The sensor parameters consisted of signals from the CAN bus, including exhaust gas temperature from the engine, pressure measurements, rotational speed, engine load, battery voltage and current, among others. The maintenance records included both planned and unplanned maintenance activities.
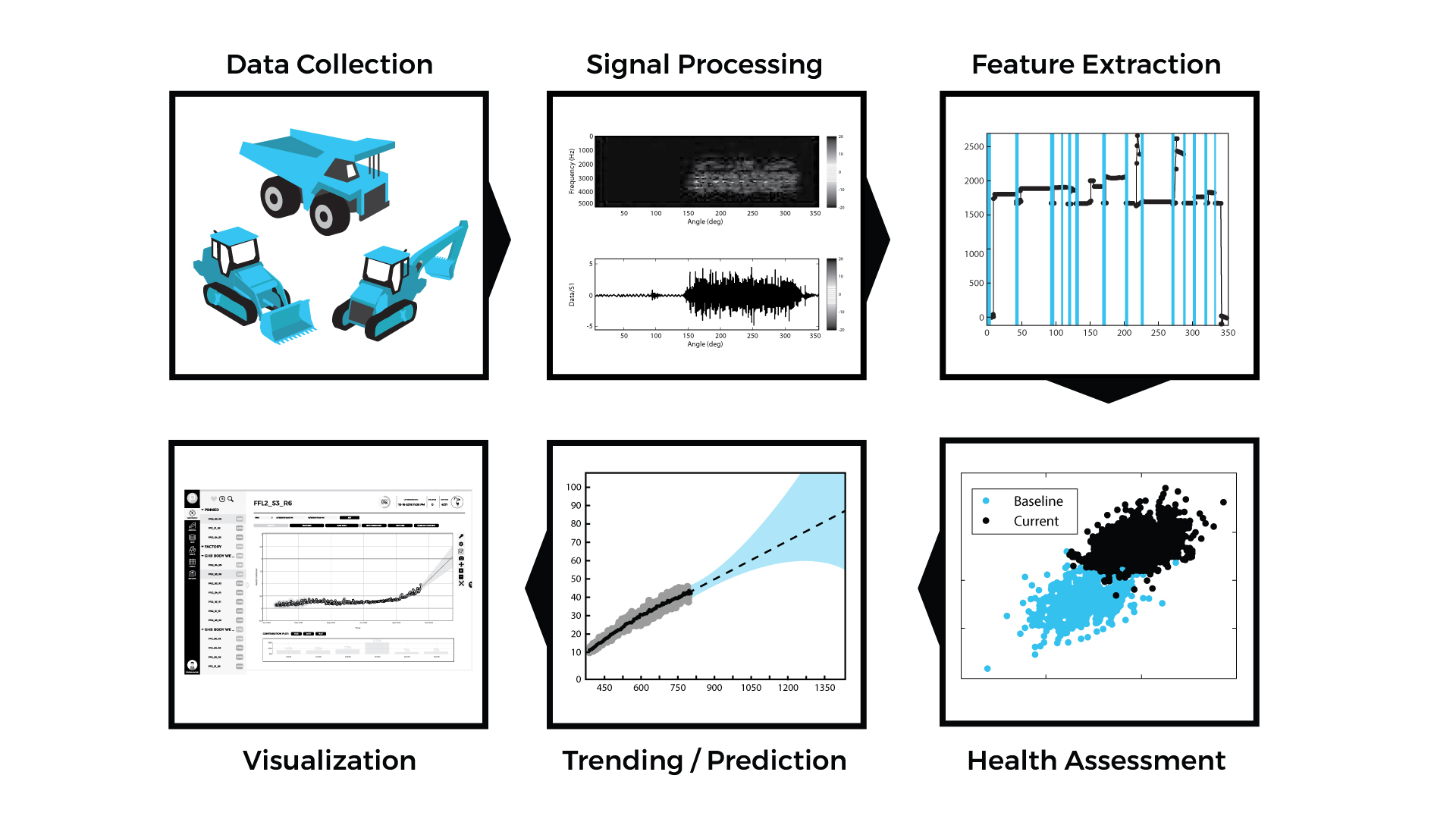
The Solution
Sensor parameters associated with the vehicle engine, transmission and electrical system (battery) were used to configure health monitoring solutions for each of these individual subsystems. This subsystem model approach was used to streamline the analysis process, as examining all 45 signals in one model would likely yield inaccurate results and make it more difficult to interpret these results and troubleshoot. Instead, it was most logical to divide the vehicle into its subsystems for health monitoring.
Machine learning was used to generate predicted parameter values, which were then compared to actual values. The predicted sensor values are based on a machine learning model that learns the baseline values and correlation relationship in the measured signals. This allowed the company to compare the temperature, pressure and other sensor readings at different vehicle operating conditions, which was more robust than simply comparing sensor values to thresholds. This residual-based analysis approach, along with the application of additional analysis methods, was the key to identifying variations in behavior indicative of an impending failure across different vehicle operating conditions.
By using a time-series anomaly detection model, the solution was able to easily predict a faulty valve issue within the vehicle before it occurred. This faulty valve was causing excessive and unusual oscillation in exhaust temperature. A time-series analysis model is useful in these specific circumstances when the anomalous patterns are less related to changes in value and correlation, but rather a change in the time-series pattern.
Outcomes
Through working with the predictive analytics company, this mining equipment manufacturer was able to identify the warning signs of failure several days before it occurred for one of its users. This early indicator allowed for maintenance personnel to schedule and conduct repairs during planned downtime, thus improving the availability of the vehicle and the overall productivity of the mining operation. With this new capability in place, the mining machine manufacturer is looking to expand this offering to its other mining users.
Getting Started With Predictive Maintenance
Criticality analysis is essential to select the vehicle or other asset for which a predictive maintenance solution can best be applied and to choose a solution that can provide the most value.
After determining the target assets, the most critical failure modes for those assets should be identified, along with any relevant maintenance records for unplanned and planned downtime.
Determine what historical data has been collected, if any, and review what data could be available from the asset’s controller.
Based on the available data and common failure modes, determine what, if any, additional sensors need to be added to the assets selected for the predictive monitoring solution.
These initial steps are essential to note when partnering with a technology provider and can help companies develop and adopt a predictive maintenance solution for their mining assets that is robust and accurate, helping these businesses achieve greater efficiency and ROI.