The pump industry depends on static laboratory test data and physical simulation models to understand expected pump performance for any given application. However, pump systems are complex, and real-world implementations may deviate significantly from pump performance curve estimates. This limitation affects pump system designers, installers and end users alike, with economic, engineering, maintenance and performance implications. Today, thanks to the increasing prevalence and usability of advanced analytical methods, companies can use sensor data to augment more traditional methods of understanding expected pump performance in real-world circumstances. Several technology trends are revolutionizing the ability to assess and understand pumping systems in field-deployed applications. These include the wide availability and declining cost of sensors, bandwidth, data storage and computing capabilities. The combination of these technologies enables the application of advanced data-driven approaches, such as machine learning, to complement traditional physics-based understanding of equipment performance. This approach enables improvements in both speed and accuracy of pump performance predictions. Ideally, machine learning models are trained on substantial data collections gathered during varying operational conditions, preferably reflecting a representative universe of potential events. For all labeled failure modes present in the historical data, models can be trained to detect and predict similar events. However, for greenfield installations or newly instrumented equipment, data is often sparse, and quality, representative, balanced and labeled data is rarely available. Particularly for pumps, it is a challenge to build analytics solutions based on limited operational data so end users have an estimated performance baseline for condition assessment.
Using Subject Matter Expertise
For decades, the pump industry has established an extensive knowledge base through standardized testing, physical simulation and tribal knowledge of an experienced workforce. Combining engineering knowledge with established machine learning techniques allows users to generalize and operationalize the factory test-performance results to serve as an improved reference for estimating the performance of real system installations. Provided that actual operational conditions are not drastically different from available test conditions, such an estimate should result in a valuable guide for optimizing performance and detecting operational failures. Consider the practical case of performance mapping for a centrifugal pump. The first step is obtaining expected and actual data points. Actual performance data points are extracted from real installed system measurements. Expected pump performance is obtained from manufacturer pump performance curves. Expected performance curves are digitized and scaled by application of pump affinity laws to a broader range of operational conditions to train a machine learning model on the extracted data set. For given operating conditions, i.e. flow rate and machine speed, the machine learning model returns the expected performance at ideal conditions.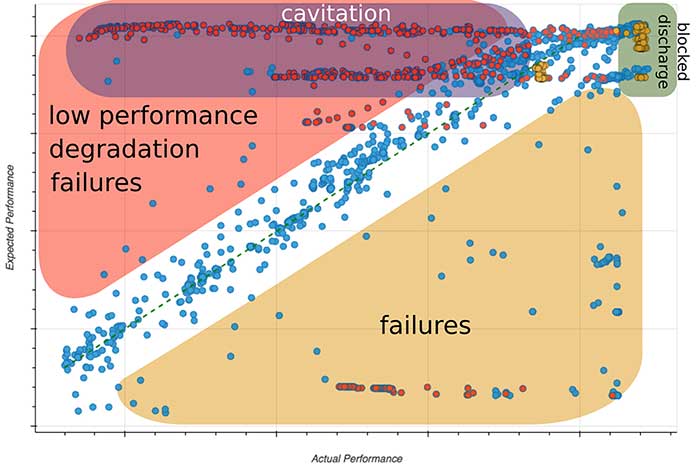