A hybrid approach can provide specialized evaluation for midtier plants and/or midcritical equipment.
Atek
06/21/2019
Imagine artificial intelligence (AI) could automate data analysis to create prescriptive and prioritized maintenance tasks. What if AI could automatically create a work order in existing computerized maintenance management systems (CMMS)? Imagine automating data collection through the internet of things (IoT) so the user experience is cost-effective and scalable. Sound too good to be true? Believe it or not, it is possible.
Where to Start
Typically, there are three major categories of conditions that lead to machinery failure:- Late-stage conditions: imbalance, misalignment, bent shaft, soft foot, looseness, etc.
- Early-stage conditions: lubrication, early-stage bearing failures
- Intermittent conditions: cavitation, resonance, speed related, process related
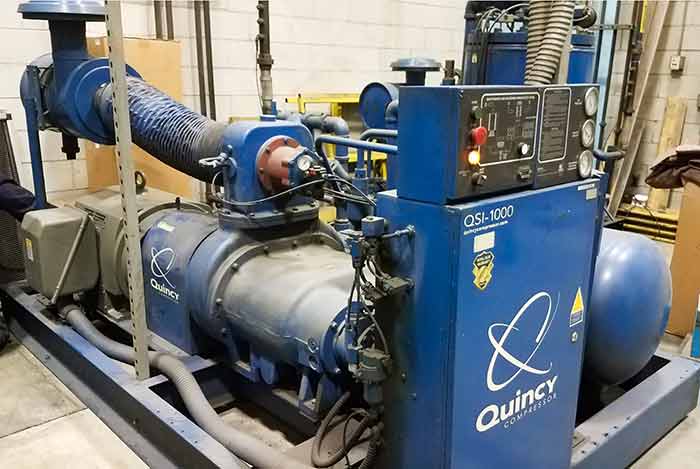
Diagnosing Early Stage Conditions
For early stage conditions, an AI model watches the frequency band from 1,000 to 30,000 Hz. This range includes ultrasonic frequencies and compares the amplitude of the ultrasonic measurement to a set of established alert limits. Ultrasonic or high-frequency monitoring is relatively new and ISO or industry standards are limited. Using experience and industry-proven alerts of 6, 12, and 18 Gs (force of gravity), the AI model sends an alert via text message or email. For example, when plant assets are operating between 600 and 6,000 rpm and a 6 Gs threshold is crossed, the automated prescriptive maintenance task is to field inspect and lubricate. Again, human experience and senses are used to collect information relating to the cause of the alert condition. Tips for the human inspection include:- asking operations about any changes or insights
- looking or smelling for smoke or hot sensations or unusual odors
- looking for leaks, spills or broken or loose supports
- listening for rubbing or grinding
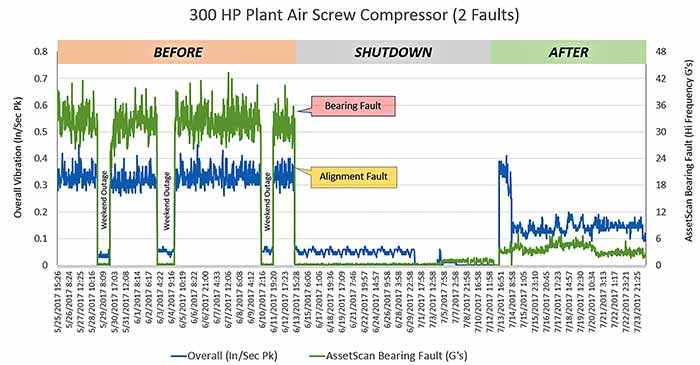
Intermittent Conditions
This is where continuous monitoring with permanent or temporary monitoring stands apart from a monthly route-based program. With wireless sensors and the IoT, coupled with the AI models described above, intermittent conditions can be monitored and root issues can be quickly identified and resolved. A common reality of vibration analysis on midcritical assets is they are typically monitored monthly, quarterly or not at all. In the route-based predictive maintenance program, the analyst gathers data monthly, taking 10 or more points per machine, and conducts a brief inspection of the machine. In contrast, continuous monitoring gathers fewer measurement points, and the data is logged and reviewed remote from the machine, in a control room, plant office, by remote third party, or all of the above. Through continuous monitoring with low-installed-cost IoT sensors, many issues that have perplexed the monthly routine are resolved. Examples vary from boiler feedwater pumps that blow a seal on the night shift, to kraft pulpers that crater a gearbox over the weekend, failed pump seals related to misalignment from thermal growth, or inconsistent lubrication practices. Intermittent issues are best solved with continuous data coupled with AI to set alert limits. These systems typically include a sensor, cellular connectivity and AI in the cloud.A 45-Day Predictive Maintenance Case Study
On May 15, a combination ultrasonic/vibration sensor was deployed to monitor a 300-horsepower (hp) plant air compressor at a Midwest industrial manufacturing plant. The sensors were battery-powered and connected to the bearings using a two-rail magnetic mount. With existing cellular, cloud and smartphone infrastructure, the hardware and system were in place. An alert system was in place with preset alert thresholds using a proven AI model. The last step was adding instruction on what to do when an alert was generated. A second AI model was used to deliver specific and customized instructions for each alert and each severity level of the alerts. This AI model translated the alert to a prescriptive maintenance task with an understanding of severity and prioritization, allowing the work to be appropriately planned and scheduled.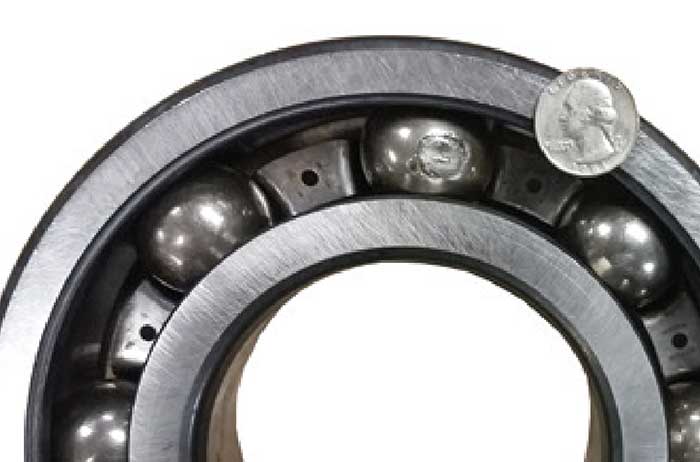