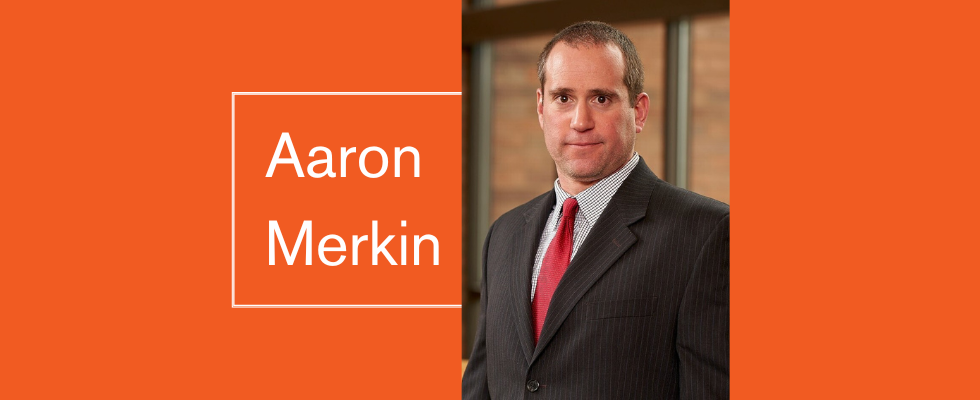
Condition monitoring data is like a trove of potential insights into machine health and plant functioning. Technicians can study vibration patterns, for example, to identify the subtle changes in machine behavior that might point to a new or developing defect. On a larger scale, condition monitoring data can be analyzed to make accurate predictions about asset failure, or about changing conditions in non-productions structural assets such as the facility itself.
But what if that data could do more? In a time when expert resources are scarce and system reliability is growing ever
more critical, artificial intelligence (AI) might hold the answers. AI technology builds on existing proactive maintenance strategies like predictive maintenance and condition monitoring.
The urgency to embrace AI is amplified by the competitive landscape. In a global economy where efficiency and reliability can be significant differentiators, early adopters of AI in condition monitoring could set the pace. These forward-thinking plants will be able to achieve operational excellence and attract talent, secure investments and lead sustainability efforts.
AI: The Missing Puzzle Piece in a Predictive Maintenance Approach
Condition monitoring is a crucial part of any predictive maintenance strategy. But on its own, condition monitoring data can’t give technicians the insights they need to transform maintenance operations.
AI tools can serve as the missing puzzle piece in a plant’s maintenance approach. When it is implemented correctly, AI can take raw data and put it to work, identifying new machine faults, diagnosing the root causes of asset failure and streamlining maintenance processes.
Again, this does not necessarily mean you need to overhaul your infrastructure. AI sits neatly on top of your condition monitoring structure, providing an extra layer of data analysis and insight.
Here is what that looks like:
Early screening and anomaly detection
AI algorithms can rapidly analyze condition monitoring data for indications an asset may be developing a new defect. One typical example use case is analyzing vibration levels for instances when the levels exceed a predetermined threshold. When vibration patterns change beyond a certain level, the algorithm registers the anomaly and issues an alert to technicians.
AI can sit on top of most data collection processes, providing an extra layer of analysis. That could mean analyzing supervisory control and data acquisition (SCADA) data on inlet/outlet pressure to identify potential issues with a pump system, for example. It could also mean analyzing building data to spot sudden changes in temperature. The potential use cases are almost limitless.
What all these use cases do have in common is that AI is reducing the drain on resources. Adding that AI layer means technicians no longer have to study every single vibration table or keep an eye on every single machine. Instead, they are notified when anomalies occur and can focus their efforts on those anomalies.
Detailed predictions and diagnostics
AI algorithms can go a few steps beyond early asset screening. Today’s AI tools can also do root cause diagnosis, identifying the source of the anomalies in condition monitoring data. That means making data-driven determinations about why there is
a change in vibration levels, for example: is it a bearing fault, a bent shaft or some other fault?
AI models can successfully diagnose these kinds of issues and can even guide technicians through the process of correcting them. When combined with generative AI capabilities, AI can give step-by-step directions, assist in troubleshooting and answer questions for operators and technicians in the field. Again, this is a case of AI extending a plant’s existing capabilities and building on what teams are already doing.
It is worth noting that if your focus is on anomaly detection for a large number of assets, AI may even be able to accomplish the task with less data than a human operator might need. If you are using AI for detailed diagnosis, you will benefit from using more data, including historical data and work orders. But in either case, the type of data needed, and the format, remain the same whether you are using AI or human technicians to carry out your analysis.
The Future Is Here & Now
The integration of AI into condition monitoring systems represents not just an evolution but a revolution in how plants operate, maintain equipment and ensure efficiency and reliability. This transformation is not a distant possibility; it is a current reality, demanding immediate action from businesses aiming to stay competitive.
As the technology landscape shifts, plants that adopt AI-driven strategies today position themselves at the forefront of innovation, setting new standards for performance, safety and sustainability. The message is clear: move quickly to adapt, or risk falling behind as the new norms and best practices for high-performing plants are established.
The adoption of AI is more than an upgrade; it is a call to action for industries to reimagine their operations. In an era where downtime is increasingly costly and efficiency is paramount, AI offers unparalleled opportunities for predictive maintenance, anomaly detection and data-driven decision-making. However, leveraging AI requires more than just technological investment; it necessitates a cultural shift within organizations.
Plants must cultivate a mindset of continuous improvement and innovation, where employees are empowered to work alongside AI, interpreting its insights and implementing its recommendations. The goal is not to replace human expertise but to augment it, creating synergies that drive performance to new heights.
Adding the AI Layer
If your plant is already streaming condition monitoring data to the cloud, then congratulations! You already have the infrastructure in place to enable AI. The more data you are collecting, the better. AI learns and can self-correct as it ingests more data.
You do not need to worry about processing and formatting all that data. AI’s automation will help with that piece. In fact, adding the AI layer will free up your team from the chore of organizing and classifying condition monitoring data.
AI may ultimately even “learn” your facility’s formatting and naming conventions to make populating databases easier and to ensure all fields are correctly filled out.
Broadly, this means as long as you are collecting condition monitoring data, you are in a good position to introduce AI to your maintenance operation. Not only can the right technology sit neatly atop your existing condition monitoring system, it will also streamline time-consuming processes and boost overall efficiency.
By the same token, if you are not collecting condition monitoring data yet, this is a great time to start. A condition monitoring and predictive maintenance strategies approach flows naturally into an AI-driven approach, so the initial investment of resources will continue to pay dividends into the future.
Asking the Right Questions About Implementing AI
It is worth stressing that AI is a means to an end rather than an end-goal. If you are considering introducing AI into your maintenance approach, make sure you are doing it for the right reasons. That means asking the right questions and setting the right goals. It also means assessing existing operations to see whether you already have the necessary infrastructure to support an AI layer.
The first step to implementing AI is building a strong condition monitoring program, with data collection as the foundation. If you are already collecting data on your assets, buildings or equipment, you are in a good position to start introducing AI. If you do not have a condition monitoring approach in place, this is the time to develop one.
Most teams will likely need to work with experts to create the AI layer and to customize the AI tools to best meet the needs of the facility. The foundational layer, though, remains the same for every facility. Collecting and analyzing condition monitoring data is a proven means of improving machine productivity and extending asset life spans.
When used correctly, AI can deepen and extend the reach of condition monitoring programs. The result? Continuous improvement and an ever better use of resources.
Seize the Opportunity
This transition to AI-enhanced operations is inevitable; the question is not if but when. Thus, the time to act is now. By embracing AI, plants commit to a future of innovation, resilience and leadership. The journey toward AI integration may be complex, but the rewards—enhanced performance, reduced costs and a sustainable competitive advantage—are too significant to ignore. The future is here and now. Let’s seize the opportunity to redefine what is possible in plant maintenance and operations.